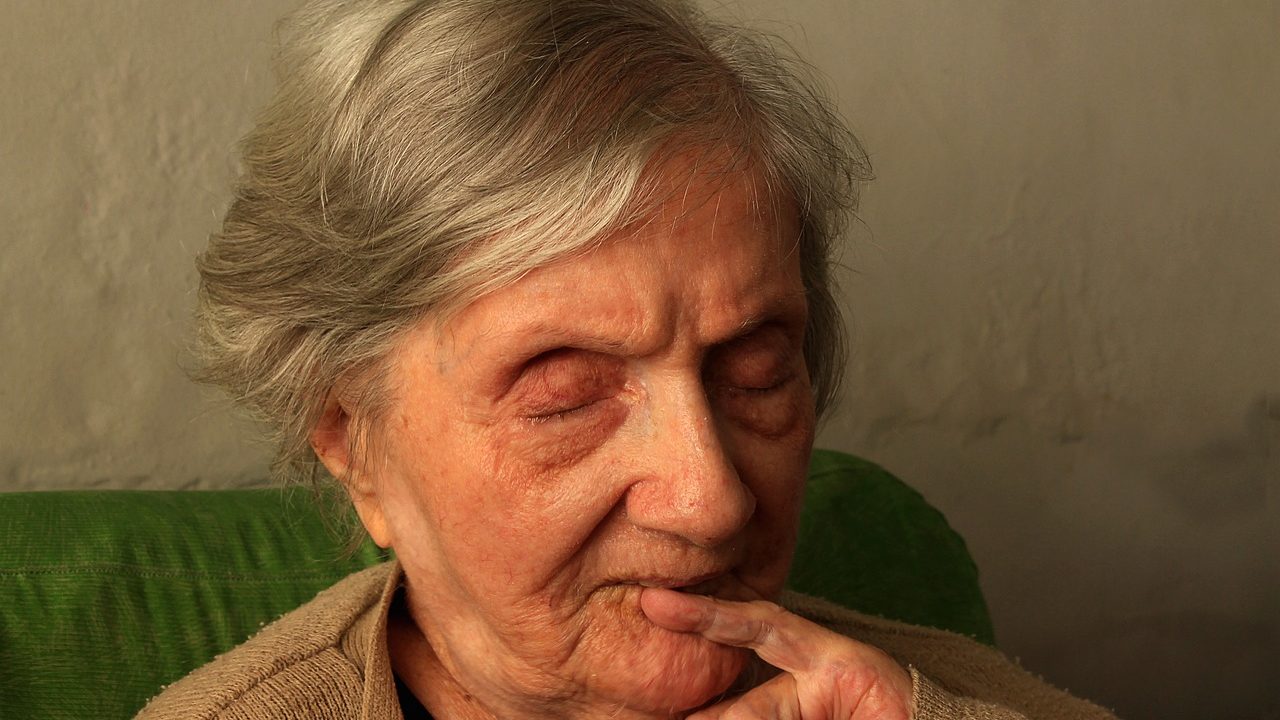
New method uses common brain imaging to detect the disease with more than 90% accuracy
Scientists at Massachusetts General Hospital (MGH) are innovating on the methods for detection of Alzheimer’s disease using artificial intelligence and deep learning. In a study published in the renowned journal PLOS ONE, the team led by Matthew Leming, PhD, a researcher at the MGH Center for Systems Biology, developed an algorithm capable of identifying the disease with 90.2% accuracy.
While previous research has focused on high-quality brain imaging scans collected in research studies, the breakthrough of this new approach lies in its application to routinely collected clinical brain imaging. This means that more accurate diagnoses can be made in the context of ordinary medical care.
The method uses deep learning, a type of artificial intelligence that uses complex algorithms and large amounts of data to train models. In this case, scientists developed a standard based on brain MRI images collected from patients with and without Alzheimer’s who were treated at MGH before 2019.
In the study, 11,103 images of 2,348 patients at risk of developing Alzheimer’s and 26,892 images of 8,456 patients without the disease were analyzed. The model was tested on five different datasets from MGH, Brigham and Women’s Hospital, and other external systems. Accuracy of 90.2% was observed across all datasets, demonstrating the algorithm’s ability to detect disease in different clinical settings and over time.
New deep learning model
In this work, scientists presented a new deep learning architecture called MUCRAN (Multi-Confound Regression Adversarial Network). It trains a deep learning model on clinical brain MRI images while regulating demographic and technical confounders.
In training MUCRAN, the researchers used 17,076 axial T1 brain MRI images collected at Massachusetts General Hospital before 2019. The new model was able to successfully reduce the main confounding factors present in this large set of clinical data. A method was also used to quantify the uncertainty of these models, which allowed us to automatically exclude data outside the distribution when detecting Alzheimer’s disease.
By combining MUCRAN with the uncertainty quantification method, consistent and significant increases in the accuracy of Alzheimer’s disease detection were observed for data recently collected from Massachusetts General Hospital (84.6% with MUCRAN versus 72.5% without MUCRAN) and also for data from other hospitals (90.3% from Brigham and Women’s Hospital and 81.0% from other hospitals).
According to the research team, MUCRAN offers a general approach for disease detection based on deep learning in heterogeneous clinical data.
The article can be accessed here.